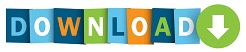
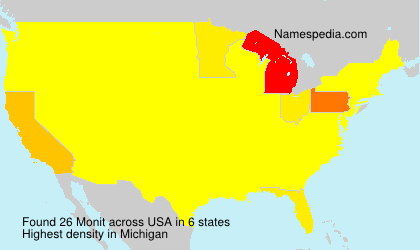
Redmon J, Divvala S, Girshick R, Farhadi A. Faster R-CNN: towards real-time object detection with region proposal networks. In: Proceedings of 2015 IEEE International Conference on Computer Vision 2015 Dec 7–13 Santiago, Chile. In: Proceedings of 2014 IEEE Conference on Computer Vision and Pattern Recognition 2014 Jun 23–28 Columbus, OH, USA. Rich feature hierarchies for accurate object detection and semantic segmentation. Girshick R, Donahue J, Darrell T, Malik J. In: Fleet D, Pajdla T, Schiele B, Tuytelaars T, editors. Spatial pyramid pooling in deep convolutional networks for visual recognition. Indoor semantic segmentation using depth information. Couprie C, Farabet C, Najman L, LeCun Y. SegNet: a deep convolutional encoder-decoder architecture for image segmentation. Learning hierarchical features for scene labeling. Farabet C, Couprie C, Najman L, LeCun Y. In: Proceedings of 2015 IEEE Conference on Computer Vision and Pattern Recognition 2015 Jun 7–12 Boston, MA, USA. Fully convolutional networks for semantic segmentation. Deep residual learning for image steganalysis. Gradient-based learning applied to document recognition. In: Proceedings of the 3rd International Conference on Learning Representations 2015 May 7–9 San Diego, CA, USA 2015. Adam: a method for stochastic optimization. Learning representations by backpropagating errors. Proceedings of the 14th International Conference on Artificial Intelligence and Statistics 2011 Apr 11–13 Fort Lauderdale, FL, USA 2011. In: Gordon G, Dunson D, Dudík M, editors. Pattern recognition and machine learning. In: Proceedings of the 15th International Conference on Pattern Recognition 2000 Sep 3–7 Barcelona, Spain. Ball tracking and virtual replays for innovative tennis broadcasts. In: Proceedings of 2005 IEEE Computer Society Conference on Computer Vision and Pattern Recognition 2005 Jun 20–25 San Diego, CA, USA. Histograms of oriented gradients for human detection. In: Proceedings of 1991 IEEE Computer Society Conference on Computer Vision and Pattern Recognition 1991 Jun 3–6 Maui, HI, USA. Computer vision: algorithms and applications. Washington, DC: United States Postal Service 1956. Postal mechanization and early automation. Cambridge: Massachusetts Institute of Technology 1963. Machine perception of three-dimensional solids. State-of-the-art in structural health monitoring of large and complex civil infrastructures. Structural health monitoring of civil infrastructure. Vibration based condition monitoring: a review. Structural health monitoring in mainland China: review and future trends. Washington, DC: US Army Corps of Engineers 2012. Corps of Engineers inspection team goes to new heights. Federal Highway Administration, Department of Transportation. Redwood City: Applied Technology Council 2005. ATC-20-1 Field manual: postearthquake safety evaluation of buildings (second edition). Reston: American Society of Civil Engineers 2017. ASCE’s 2017 infrastructure report card: bridges. The paper concludes with ongoing work aimed at addressing some of these stated challenges. Subsequently, some of the key challenges that persist towards the goal of automated vision-based civil infrastructure and monitoring are presented. The monitoring applications discussed include static measurement of strain and displacement, as well as dynamic measurement of displacement for modal analysis. The inspection applications reviewed include identifying context such as structural components, characterizing local and global visible damage, and detecting changes from a reference image. The work reviewed is classified into two types: inspection applications and monitoring applications. In particular, relevant research in the fields of computer vision, machine learning, and structural engineering are presented. This paper provides an overview of recent advances in computer vision techniques as they apply to the problem of civil infrastructure condition assessment. The ultimate goal of such a system is to automatically and robustly convert the image or video data into actionable information. Computer vision techniques, in conjunction with acquisition through remote cameras and unmanned aerial vehicles (UAVs), offer promising non-contact solutions to civil infrastructure condition assessment.
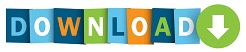